Key Takeaways:
- AI is reshaping drug discovery, reducing timelines and costs through powerful data-driven algorithms.
- Tools like machine learning and predictive modeling are identifying targets and optimizing clinical trials.
- Biotech companies adopting AI see faster development pipelines and higher success rates in delivering therapies.
The traditional drug discovery process is lengthy, expensive, and fraught with uncertainty. On average, it takes over a decade and costs upwards of $1 billion to bring a new drug to market. However, artificial intelligence (AI) is changing the game by streamlining this process, improving accuracy, and opening doors to breakthroughs that once seemed out of reach.
From identifying novel drug targets to predicting patient responses in clinical trials, AI is becoming a cornerstone of biotech innovation. Companies and researchers are leveraging these tools to develop life-changing therapies faster and more efficiently.
How AI is Revolutionizing Drug Discovery
Target Identification and Validation
AI can process vast datasets, such as genomic and proteomic information, to pinpoint drug targets linked to specific diseases.- Example: AI-driven platforms like DeepMind’s AlphaFold predict protein structures, helping researchers identify binding sites for drug candidates.
Drug Design and Optimization
Machine learning algorithms analyze chemical libraries to design molecules with optimal therapeutic properties.- AI tools reduce the trial-and-error process, creating drug candidates that are more likely to succeed in preclinical studies.
Repurposing Existing Drugs
AI algorithms identify new applications for approved drugs or those in development pipelines, saving years of research time.- Example: BenevolentAI used AI to repurpose baricitinib for treating COVID-19.
Streamlining Clinical Trials
AI predicts patient responses, stratifies populations, and identifies optimal trial sites, reducing the risk of failure in late-stage trials.- AI-enabled tools from companies like Exscientia ensure that trials are more efficient and cost-effective.
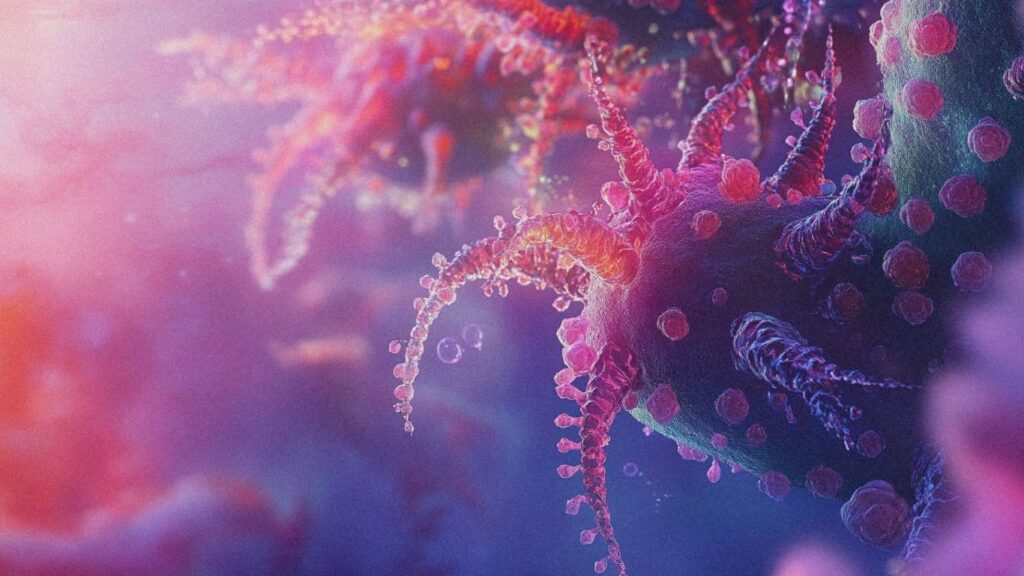
The Benefits of AI in Drug Discovery
- Speed: AI significantly reduces the time required to identify promising drug candidates, accelerating early research phases.
- Cost Efficiency: By eliminating less viable options early in the pipeline, AI reduces the financial burden of failed trials.
- Improved Precision: Predictive models improve accuracy in selecting drug targets and identifying patient populations.
- Broader Applications: AI can explore complex disease mechanisms and identify therapies for conditions that were previously too challenging to address.
Challenges of AI Adoption in Biotech
While AI offers transformative potential, implementing it isn’t without hurdles:
- Data Quality and Access: AI models require high-quality, diverse datasets to deliver meaningful insights, but such data can be hard to obtain or siloed across organizations.
- Regulatory Frameworks: Current regulations are not always equipped to handle AI-driven drug discovery, leading to delays in approval.
- High Initial Investment: AI systems require substantial upfront costs for implementation and integration.
Despite these challenges, the rewards of AI adoption in drug discovery far outweigh the risks, especially as companies refine their tools and practices.
Key Players in AI-Driven Drug Discovery
Several companies are leading the charge in integrating AI into drug discovery:
- Exscientia: Pioneers in AI-designed precision drugs, with multiple candidates already in clinical trials.
- Insilico Medicine: Uses AI to identify novel drug candidates and predict their success in preclinical stages.
- DeepMind (Google): Revolutionized protein folding predictions, aiding researchers in understanding disease mechanisms.
- Schrödinger: Combines AI with physics-based modeling to optimize drug discovery.
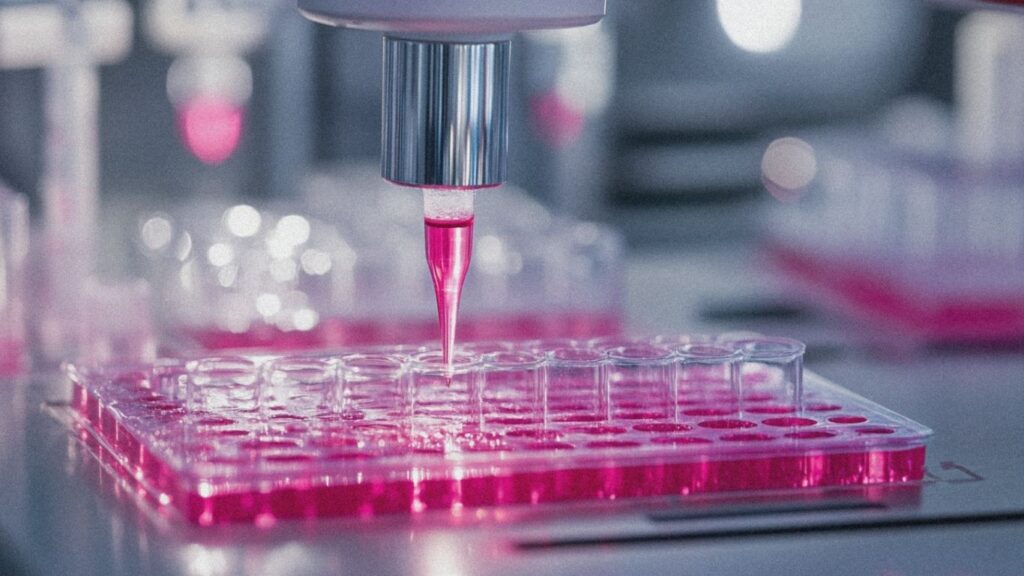
Real-World Impact: Case Studies
DeepMind’s AlphaFold
In 2021, AlphaFold provided near-complete protein structure predictions for the human proteome, accelerating drug discovery efforts for diseases like Alzheimer’s and cancer.Exscientia’s AI-Driven Pipeline
Exscientia developed a drug candidate for obsessive-compulsive disorder (OCD) in under a year, a process that typically takes 4–5 years.Insilico Medicine’s Breakthrough
In 2020, Insilico Medicine identified a preclinical candidate for idiopathic pulmonary fibrosis in just 18 months, showcasing AI’s ability to significantly shorten timelines.
The Future of AI in Drug Discovery
As AI continues to mature, its applications in drug discovery will only expand:
- Personalized Medicine: AI tools will help tailor therapies to individual patients based on their unique genetic and phenotypic data.
- Improved Decision-Making: Predictive modeling will make early-stage research more accurate and efficient, reducing the likelihood of late-stage trial failures.
- Wider Adoption: As the cost of AI tools decreases, more biotech companies will integrate them into their workflows, democratizing innovation across the industry.
Conclusion
The AI revolution in drug discovery is here, and it’s accelerating the development of therapies that could change lives. By reducing time-to-market, improving accuracy, and lowering costs, AI is creating a more efficient and innovative biotech landscape. As companies like Exscientia, Insilico Medicine, and DeepMind continue to push the boundaries, the future of drug discovery looks brighter than ever.
For biotech professionals, understanding and adopting AI tools isn’t just an option—it’s a necessity to stay competitive in a rapidly evolving industry.